Experimentally determining what a protein looks like is a time consuming process that can extend for months or even years; it often also comes with a hefty price tag. This is why, for decades, researchers have been actively engaged in the pursuit of computational methods to predict a protein’s three-dimensional structure from its amino acid sequence.
The problem has been a tough nut to crack.
Demis Hassabis cofounded DeepMind and is one of the leaders of the AlphaFold project.
Credit: DeepMind
In 2020, Google DeepMind took a giant leap in this regard. In the biennial Critical Assessment of Structure Prediction competition, contest organizers present experts with amino acid sequences of proteins with a known but unpublished structure. AlphaFold2, an improved version of the very first AlphaFold, achieved results that significantly outperformed all other entrants in 2020 and previous years (1). Using deep learning methods, the technology made extremely accurate predictions for a protein in a matter of minutes.
For their leadership in developing AlphaFold, Demis Hassabis and John Jumper from DeepMind Google UK received the 2023 Albert Lasker Basic Medical Research Award.
“What [AlphaFold] has managed to do is develop a much more sophisticated . . . understanding of the rules that are driving the 3D fold,” said Bissan Al-Lazikani, a data scientist and drug discovery researcher at the MD Anderson Cancer Center.
The use of deep learning in AlphaFold enabled researchers to move away from basic physics-based presumptions that may not always offer the best path to prediction, Al-Lazikani said. “The nice thing about the AlphaFold algorithm is it almost liberated itself from [those] constraints,” she added, “and clearly has done a phenomenal job.”
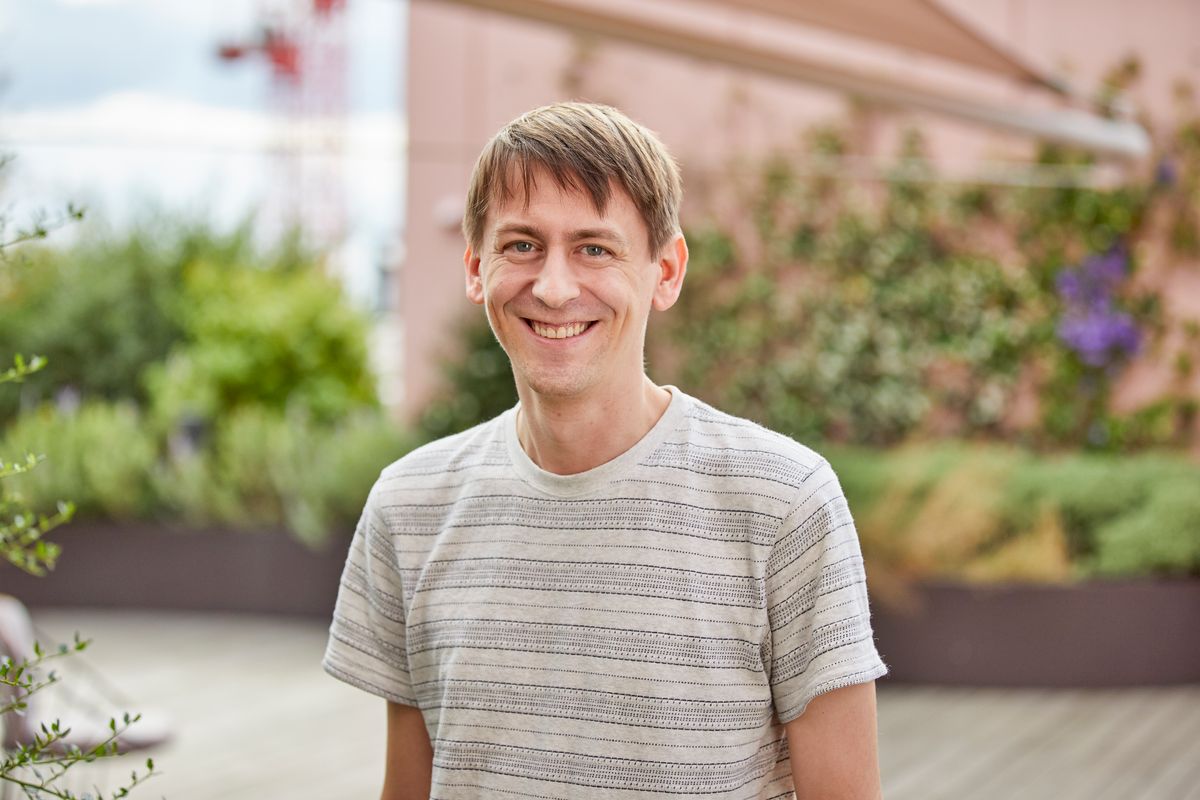
John Jumper is one of the leaders of the AlphaFold project, which resulted in an algorithm that predicts protein structure using deep learning methods.
Credit: DeepMind
Since both the AlphaFold protocol and a database containing the million protein structure predictions obtained from the software are publicly available, multiple research teams have been using it to study proteins relevant to biomedical research (2). “A really important use for it has been in trying to probe, even at a rough level, whether structures are druggable,” said Al-Lazikani. For instance, researchers can see whether they can design a drug to fit into a specific cavity in the protein structure. “Although it’s not perfect, at least we can do that, and we can do that at a scale now that we couldn’t before,” she said.
“It’s been an enormous team effort by scientists over the last 50 years,” said Alex Bateman, an AlphaFold team member and a computational biologist at the European Bioinformatics Institute-European Molecular Biology Laboratory. He added that the DeepMind team nicely brought together ideas that have been developed throughout the decades within this deep-learning framework.
“The contributions of DeepMind and Demis and John are really spectacular,” Bateman said. “They broke this mental barrier that you really can’t solve the structure of a protein computationally at very high accuracy.”
References
- Tunyasuvunakool, K. et al. Highly accurate protein structure prediction for the human proteome. Nature 596, 590-596.
- AlphaFold Protein Structure Database. At https://alphafold.ebi.ac.uk/
Credit: Source link