For large scale Generative AI application to work well, it needs good system to handle a lot of data. One such important system is the vector database. This database is special because it deals with many types of data like text, sound, pictures, and videos in a number/vector form.
What are Vector Databases?
Vector database is a specialized storage system designed to handle high-dimensional vectors efficiently. These vectors, which can be thought of as points in a multi-dimensional space, often represent embeddings or compressed representations of more complex data like images, text, or sound. Vector databases allow for rapid similarity searches amongst these vectors, enabling quick retrieval of the most similar items from a vast dataset.
Traditional Databases vs. Vector Databases
Vector Databases:
- Handles High-Dimensional Data: Vector databases are designed to manage and store data in high-dimensional spaces. This is particularly useful for applications like machine learning, where data points (such as images or text) can be represented as vectors in multi-dimensional spaces.
- Optimized for Similarity Search: One standout features of vector databases is their ability to perform similarity searches. Instead of querying data based on exact matches, these databases allow users to retrieve data that is “similar” to a given query, making them invaluable for tasks like image or text retrieval.
- Scalable for Large Datasets: As AI and machine learning applications continue to grow, so does the amount of data they process. Vector databases are built to scale, ensuring that they can handle vast amounts of data without compromising on performance.
Traditional Databases:
- Structured Data Storage: Traditional databases, like relational databases, are designed to store structured data. This means data is organized into predefined tables, rows, and columns, ensuring data integrity and consistency.
- Optimized for CRUD Operations: Traditional databases are primarily optimized for CRUD operations. This means they are designed to efficiently create, read, update, and delete data entries, making them suitable for a wide range of applications, from web services to enterprise software.
- Fixed Schema: One of the defining characteristics of many traditional databases is their fixed schema. Once the database structure is defined, making changes can be complex and time-consuming. This rigidity ensures data consistency but can be less flexible than the schema-less or dynamic schema nature of some modern databases.
Old databases struggle with embeddings. They can’t handle their complexity. Vector databases solve this problem.
With vector databases, Generative AI application can do more things. It can find information based on meaning and remember things for a long time.
Vector Database

High-Level Architecture of a Vector Database
The diagram shows the fundamental workflow of a vector database. The process begins with raw data input, which undergoes preprocessing to clean and standardize the data.
This data is then vectorized, converting it into a format suitable for similarity searches and efficient storage. Once vectorized, the data is stored and indexed to facilitate rapid and accurate retrieval. When a query is made, the database processes it, leveraging the indexing to efficiently retrieve the most relevant data.
Generative AI and The Need for Vector Databases
Generative AI often involves embeddings. Take, for instance, word embeddings in natural language processing (NLP). Words or sentences are transformed into vectors that capture semantic meaning. When generating human-like text, models need to rapidly compare and retrieve relevant embeddings, ensuring that the generated text maintains contextual meanings.
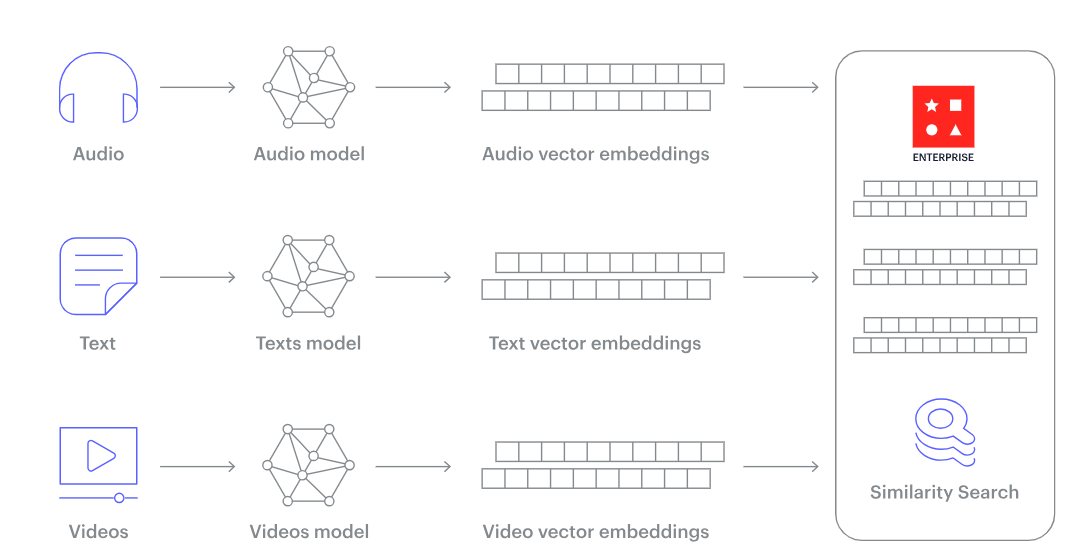
Vector Database redis db
Similarly, in image or sound generation, embeddings play a crucial role in encoding patterns and features. For these models to function optimally, they require a database that allows for instantaneous retrieval of similar vectors, making vector databases an essential component of the generative AI puzzle.
Creating embeddings for natural language usually involves using pre-trained models such as OpenAI’s GPT, BERT.
Pre-trained Models:
- GPT-3 and GPT-4: OpenAI’s GPT-3 (Generative Pre-trained Transformer 3) has been a monumental model in the NLP community with 175 billion parameters. Following it, GPT-4, with an even larger number of parameters, continues to push the boundaries in generating high-quality embeddings. These models are trained on diverse datasets, enabling them to create embeddings that capture a wide array of linguistic nuances.
- BERT and its Variants: BERT (Bidirectional Encoder Representations from Transformers) by Google, is another significant model that has seen various updates and iterations like RoBERTa, and DistillBERT. BERT’s bidirectional training, which reads text in both directions, is particularly adept at understanding the context surrounding a word.
- ELECTRA: A more recent model that is efficient and performs at par with much larger models like GPT-3 and BERT while requiring less computing resources. ELECTRA discriminates between real and fake data during pre-training, which helps in generating more refined embeddings.
Growing Funding for Vector Database Newcomers
With AI’s rising popularity, many companies are putting more money into vector databases to make their algorithms better and faster. This can be seen with the recent investments in vector database startups like Pinecone, Chroma DB, and Weviate.
Large cooperation like Microsoft have their own tools too. For example, Azure Cognitive Search lets businesses create AI tools using vector databases.
Oracle also recently announced new features for its Database 23c, introducing an Integrated Vector Database. Named “AI Vector Search,” it will have a new data type, indexes, and search tools to store and search through data like documents and images using vectors. It supports Retrieval Augmented Generation (RAG), which combines large language models with business data for better answers to language questions without sharing private data.
Primary Considerations of Vector Databases
- Indexing: Given the high-dimensionality of vectors, traditional indexing methods don’t cut it. Vector databases uses techniques like Hierarchical Navigable Small World (HNSW) graphs or Annoy trees, allowing for efficient partitioning of the vector space and rapid nearest-neighbor searches.
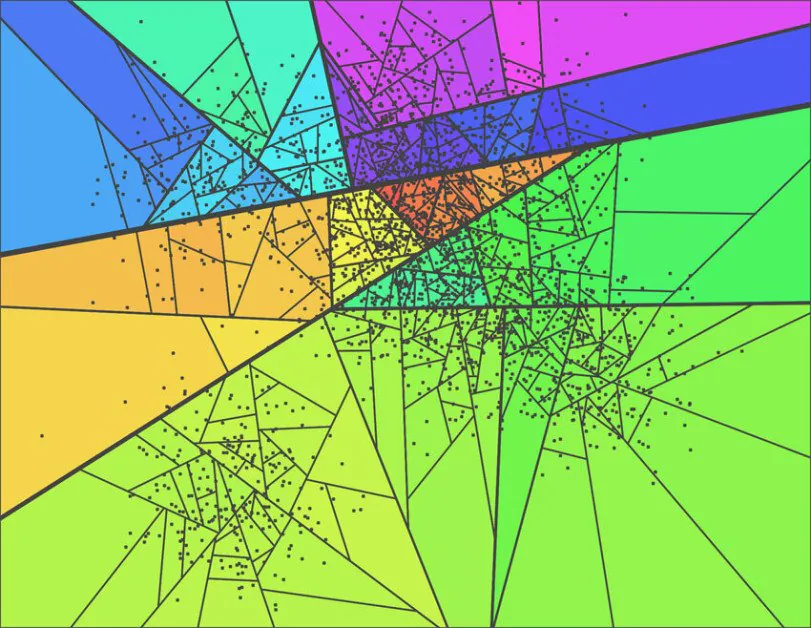
Annoy tree (Source)
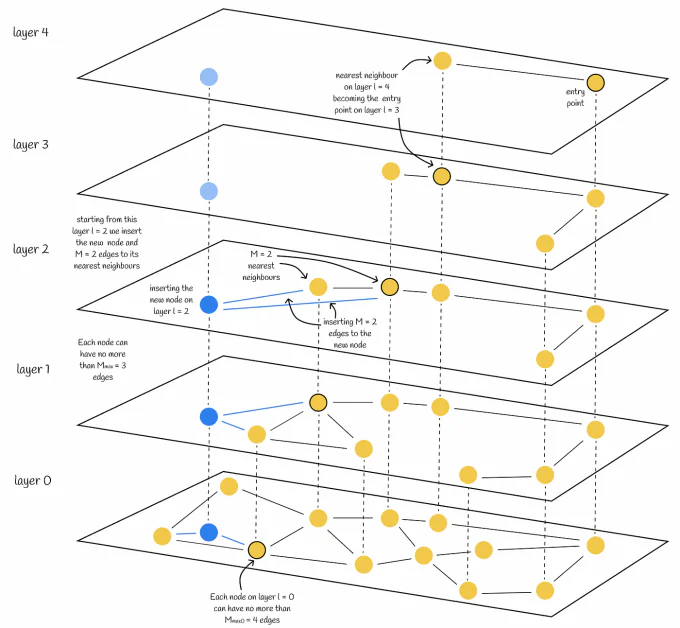
Hierarchical Navigable Small World (HNSW) graphs (Source)
- Distance Metrics: The effectiveness of a similarity search hinges on the chosen distance metric. Common metrics include Euclidean distance and cosine similarity, each catering to different types of vector distributions.
- Scalability: As datasets grow, so does the challenge of maintaining fast retrieval times. Distributed systems, GPU acceleration, and optimized memory management are some ways vector databases tackle scalability.
Vector Databases and Generative AI: Speed and Creativity
The real magic unfolds when vector databases work in tandem with generative AI models. Here’s why:
- Enhanced Coherence: By enabling rapid retrieval of similar vectors, generative models can maintain better context, leading to more coherent and contextually appropriate outputs.
- Iterative Refinement: Generative models can use vector databases to compare generated outputs against a repository of ‘good’ embeddings, allowing them to refine their outputs in real-time.
- Diverse Outputs: With the ability to explore various regions of the vector space, generative models can produce a wider variety of outputs, enriching their creative potential.
The Future: Potential Implications and Opportunities
With the convergence of generative AI and vector databases, several exciting possibilities emerge:
- Personalized Content Creation: Imagine AI models tailoring content, be it text, images, or music, based on individual user embeddings stored in vector databases. The era of hyper-personalized content might not be far off.
- Advanced Data Retrieval: Beyond generative AI, vector databases can revolutionize data retrieval in domains like e-commerce, where product recommendations could be based on deep embeddings rather than superficial tags.
The AI world is changing fast. It’s touching many industries, bringing good things and new problems. AI now needs good data processing. This is because of big language models, generative AI, and semantic search.
Credit: Source link